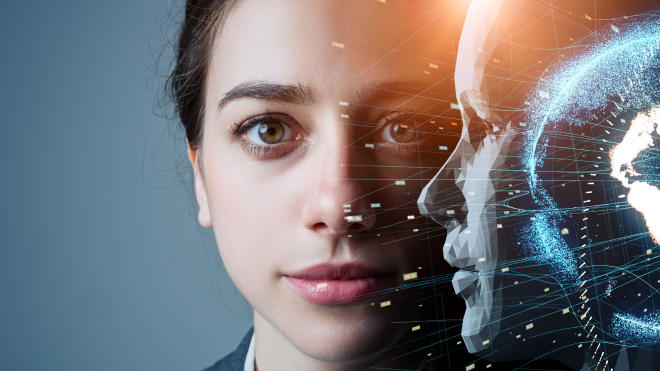
14 Nov Machine learning automation is a necessary step in the development of AI as a Service
Artificial intelligence systems continue to capture the consumer services market at great speed. Many state enterprises, private organizations and ordinary consumers seek to use the advantages for their own development. However, there are still a number of reasons that significantly hinder the widespread introduction of artificial intelligence into society. One of them is the difficulty of building a machine learning model for the tasks.
Building a machine learning model is an iterative process. Machine learning models are built into six steps: data access and collection, data preparation and exploration, model build and train, model evaluation, model deployment and model monitoring. This requires a lot of labour and financial investments, which may not be available to every consumer.
The solution to this problem could be automating machine learning (AML), as many of the steps needed to build a machine learning model are reiterated and modified until data scientists are satisfied with the model performance. Many AIaaS platform vendors are already using automation to increase the availability of their services. AML can improve the productivity of data scientists by automating the training process. It also allows data analysts and developers to build machine learning models without tweaking every aspect of the model training process that comes with data science expertise. Most AML capabilities support tabular data for classification and regression problems while others have more advanced offerings that support images and text data, as well as time series forecasting.
However, the principle of automation itself requires significant refinement and development. In most cases, at the moment, machine learning automation is implemented according to the “black box” principle, when there is no clear understanding of how the resulting solution was obtained. First of all, this reduces the trustworthy of the results obtained.
Detailed study of the stages of the machine learning process, unification of the main components of the trained model, development of learning algorithms, accumulation of more data for analysis (including through artificial intelligence systems) will improve the possibilities of machine learning automation and increase the applicability of AIaaS.
Reference:
ORACLE Lifecycle of machine learning models
Author: Tetyana SOLIANYK
Links